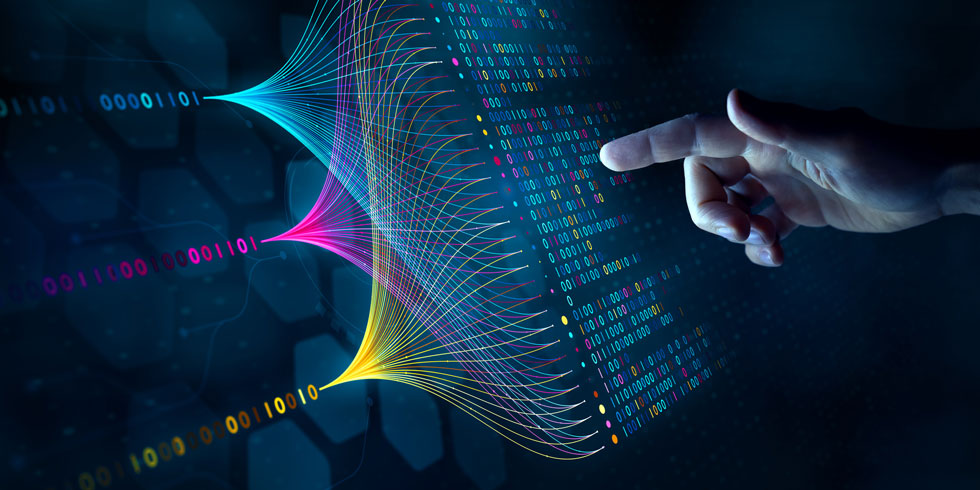
Image: iStockphoto.com/NicoElNino
Invoking AI for diagnosis: Art at the cutting edge of science
Authors: Pulikottil Wilson Vinny
Journal of the Neurological Sciences. REVIEW ARTICLE|VOLUME 453, 120803, OCTOBER 15, 2023
DOI: https://doi.org/10.1016/j.jns.2023.120803
Hazarding a neurological diagnosis has traditionally been an exercise rooted in time tested clinical methods of history taking, careful examination and neurological localization. So sacred are these tenets established by Charcot that, even in present times, a small deviation from the established path is bound to raise eyebrows. Adherence to the sanctity of the process is however no guarantee against diagnostic errors. Art must mix with science in the right proportions to get the diagnosis right. It is indeed a gift that all neurologists earn through experience. Diagnostic accuracy has been shown to vary widely among neurologists.
Deep learning analysis of UPLC-MS/MS-based metabolomics data to predict Alzheimer's disease
Authors: Kesheng Wang, Laurie A. Theeke, Christopher Liao, Nianyang Wang, Yongke Lu, Danqing Xiao, Chun Xu
Journal of the Neurological Sciences. REVIEW ARTICLE|VOLUME 453, 120812, OCTOBER 15, 2023
DOI: https://doi.org/10.1016/j.jns.2023.120812
Highlights
- Performed feature selection using LASSO and identified 21 metabolomic biomarkers.
- The highest accuracy deep learning (DL) model had two layers and 18 neurons.
- Identified metabolites are correlated with clinical CSF biomarkers (Aβ42, tTau, and pTau).
- DL can inform early diagnosis, prognostic risk stratification, and/or early interventions.
Metabolic biomarkers can potentially inform disease progression in Alzheimer's disease (AD). The purpose of this study was to identify and describe a new set of diagnostic biomarkers for developing deep learning (DL) tools to predict AD using Ultra Performance Liquid Chromatography Mass Spectrometry (UPLC-MS/MS)-based metabolomics data.
A total of 177 individuals, including 78 with AD and 99 with cognitive normal (CN), were selected from the Alzheimer's Disease Neuroimaging Initiative (ADNI) cohort along with 150 metabolomic biomarkers. We performed feature selection using the Least Absolute Shrinkage and Selection Operator (LASSO). The H2O DL function was used to build multilayer feedforward neural networks to predict AD.
This study identified a new set of diagnostic metabolomic biomarkers for developing DL tools to predict AD. These biomarkers may help with early diagnosis, prognostic risk stratification, and/or early treatment interventions for patients at risk for AD.